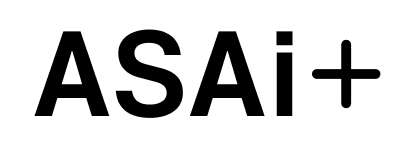
Maximize ROAS
with AI-Powered
Apple Search Ads
AI-based bid optimization
Keyword Prioritization
Continuously analyze and rank keywords to maximize relevance and performance.
Bid Optimization
Use advanced machine learning models to find the optimum bid for all your keywords.
Dynamic Budget Adjustments
Adapt and optimize your budgets in real time for maximum impact.
Designed for Next-Gen ASA Campaigns.
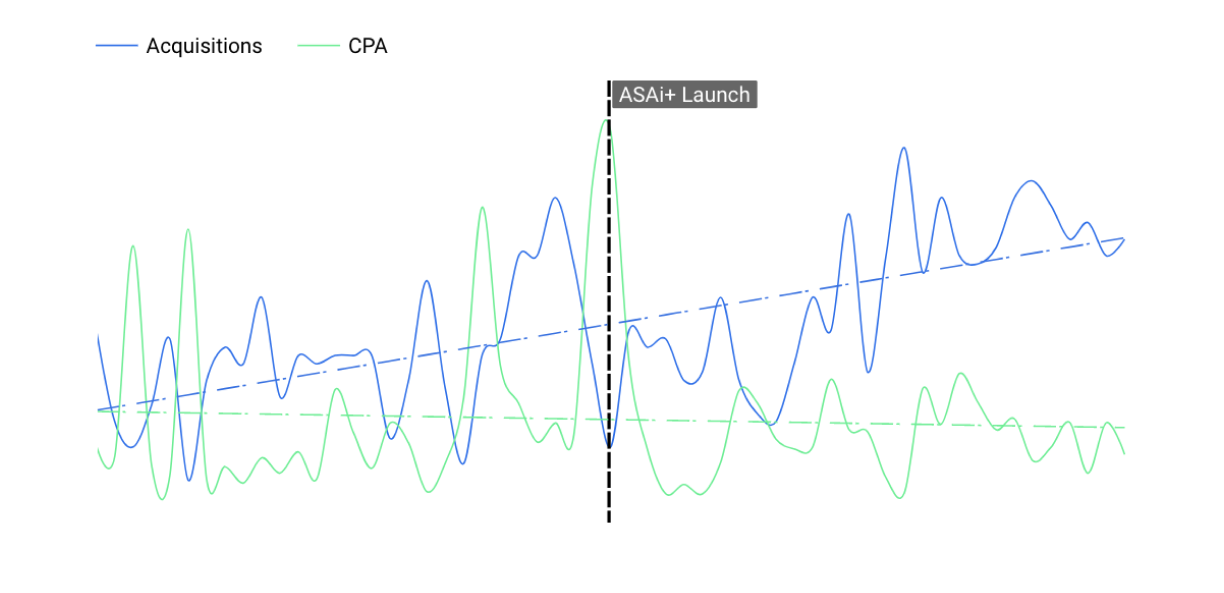
A highly adaptive,
AI-driven solution for
Apple Search Ads.
Trusted by
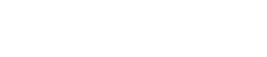
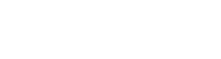
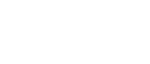
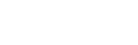
and many more
Case Study
Optimizing onX’ Apple Search Ads Bids With AI
Achieving a 20% increase in installs, while reducing CPA by 2.2%.
What you get
Reinforcement Learning
Proprietary models dynamically adjust keyword bids based on real-time market data.
Simulated Environment for Training
A simulated auction environment (ASASim) trains the models before real-world deployment.
Dynamic Budget Adjustments
ASAi+ models competitor behavior during simulations, and supports dynamic budget adjustments.
Pre-Trained Keyword Clustering
Pre-trained keyword clusters based on historical performance for tailor-made strategies.
Data Privacy
Simulated training data ensures full anonymity, meaning none of your real data is used to train our models.
Automatic Keyword Discovery & Probing
Enhanced targeting by automatically discovering new keywords and probing them for value.
Advanced Bid Exploration
ASAi+ features bid exploration to find the optimal bids, escaping the limits of preset rules.
ASAi+ vs. Other Systems
Feature | ASAi+ by Phiture | Other Systems |
---|---|---|
Reinforcement Learning (RL) | ✅ Utilizes RL to dynamically adjust keyword bids based on real-time market data for optimal performance. | 🟡 Uses predictive modeling or rule-based bidding. |
Simulated Environment & Keyword Clustering | ✅ Employs a simulated auction environment and uses pre-trained keyword clusters for accurate training and optimization. | ❌ No campaign simulation capabilities; does not emphasize pre-trained clusters for optimization. |
Dynamic Budget Adjustments & Optimization | ✅ Supports dynamic budget adjustments with automated bidding, leveraging RL to optimize budget allocation within CPA and budget targets. | 🟡 Uses predictive models for budget allocation but lacks dynamic adjustment features. |
Automatic Keyword Discovery & Probing | ✅ Automatically discovers and tests new keywords to enhance targeting and selection. | 🟡 Offers suggested keywords but lacks automatic testing and probing features. |
Adaptive Campaign Strategies | ✅ Classifies campaigns into focus states for targeted strategies and improved performance. | ❌ Does not offer campaign state analysis or adaptive campaign strategies. |
Data Privacy | ✅ Trains models using data specific to the app we're working on, ensuring no data from other apps is used. | 🟡 Aggregates and anonymizes data across multiple apps for model training. |
Performance Reporting | ✅ Offers detailed analytics and KPI reports. | ✅ Offers detailed analytics and KPI reports. |
Let's talk →
Got you interested?
Frequently asked questions
Yes, there is a monitoring dashboard which shows all keywords you are bidding on, with labels for “prioritized”, “paused” and “removed”.
Brand campaigns with enough impressions usually start seeing trends change around the two week mark. For other campaigns this might vary. After around four weeks, you should be seeing changes in performance.
ASAi+ is built around on “installs” as a KPI, but lower-funnel events can also be optimized towards — think: Purchase, subscription, etc. However, there needs to be sufficient data for this to work.
The reinforcement learning algorithms will pick up on these factors very quickly and adapt strategies accordingly.
The models utilize historical performance data in existing campaigns to speed up the training phase. If no data exists because the campaign is new, the model will start to explore bids and collects data on its own.